OBJECTIVITY/ The Subjectivity of AI
As getting access to information without the aid of an algorithm has become practically unthinkable, it is no wonder that algorithms are deeply implicated with what makes up our knowledge and truths. On a parallel note, as now somewhat commonplace phrases like ‘algorithmic governance’ imply, the same knowledge-base informs (often automated) decision-making processes. With our notion of truth tightly interwoven with the concept of objectivity, it is little surprise that algorithmic objectivity has become one of the key concerns in today’s discussions around artificial intelligence. In this essay, I aim to think beyond the issue of algorithmic objectivity, which is most often dismissed as a myth.
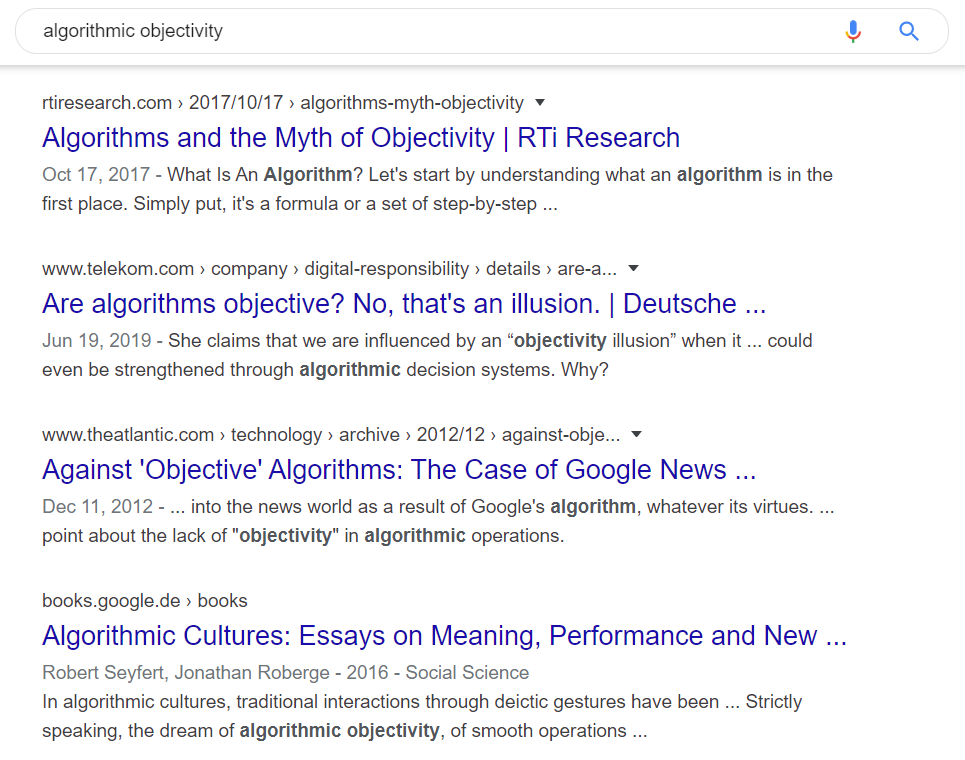
Departing from a discussion on the objectivity of algorithms, in the first section of this essay I make the argument that the myth-ness of objectivity is the dominant discourse when it comes to algorithmic objectivity. The question this poses of course, is what are algorithms, if not objective? While there are many propositions that address this question with varying levels of focus on the issue that objectivity poses, the most direct response tends to make a case for algorithmic bias. With this essay, I would like to explore an alternative to jumping to bias as the default antonym to algorithmic objectivity; namely subjectivity, as ‘bias’, in my view, primarily relays criticism for lacking objectivity to others outside itself (for example a network’s training data), and reduces the nuance/ dimensionality of objectivity, the concept it is intended to oppose. In exploring this alternative, I first discuss the works of scholars like Rouvroy (2016) and Fisher (2020), whose work deals with the influence of digital media on subjectivity. While their primary argument is that algorithms have a transformational effect on our epistemology to the extent that subjectivity can be by-passed, or made altogether obsolete, I would like to suggest that the issue could rather be one of outsourcing what was originally ‘our’ subjectivity to algorithmic agents. I draw on Fisher’s analysis of Google’s near-future algorithmic imaginary to make this point, and finally briefly discuss some of the challenges that this poses for future elaboration of the concept.
The myth of objectivity
In the past few years, inquiries into the ‘myth’ of objectivity have made discussions about the topic of algorithmic objectivity surface. This myth associated with issues of bias, discrimination or reinforcement of existing forms of suppression has become a topic of public discussion – arguably larger than explicit discourse pitching algorithmic objectivity has ever been. In other words, public discourses on algorithmic objectivity are primarily concerned with its myth. In this context, a public case for algorithmic objectivity requires qualification – requires the one making it, to acknowledge the associated contestation.
The assumption of objectivity is perhaps best seen as a default, useful to and encouraged by some, until the issue of algorithmic objectivity is put into question. The argument that a semblance of objectivity is crucial to establishing the legitimacy of artificial intelligences as actors entitled to doing the work they do is repeated across literature that critically engages with algorithmic agents (for example Morozov, 2011; Kitchin & Dodge, 2011; Gillespie, 2014; Beer, 2017). Speaking about information algorithms, Gillespie captures the general principle of this argument eloquently in stating that ‘[t]he performance of algorithmic objectivity has become fundamental to the maintenance of these tools as legitimate brokers of relevant knowledge’ (2014: pp).
Addressing this criticism has become the concern of researchers and practitioners working in the computer sciences. In 2014 Gillespie wrote that the computer sciences focus primarily on the optimisation of algorithms, with a view to their efficiency and implementation, neglecting their ‘application of circulation’. Algorithms were said to be ‘framed as objective, rational agents’ (Gillepsie, 2014: pp). Since then, this so-called ‘carefully crafted fiction’ is being openly addressed in the computer sciences, as researchers and developers try to find ‘solutions’ to the lagging objectivity of their products and tools. ‘Bias and Inclusion’ is one of the four key research themes of the AI Now Research Institute, along with ‘rights and liberties’, ‘labor and automation’, and ‘safety and critical infrastructure’. In an article entitled ‘Algorithmic Solutions to Algorithmic Bias: A Technical Guide’ published on the popular towards data science blog, Xu (2019) provides an overview of some of the practical approaches to overcoming algorithmic bias in data, specifically in the context of machine learning.
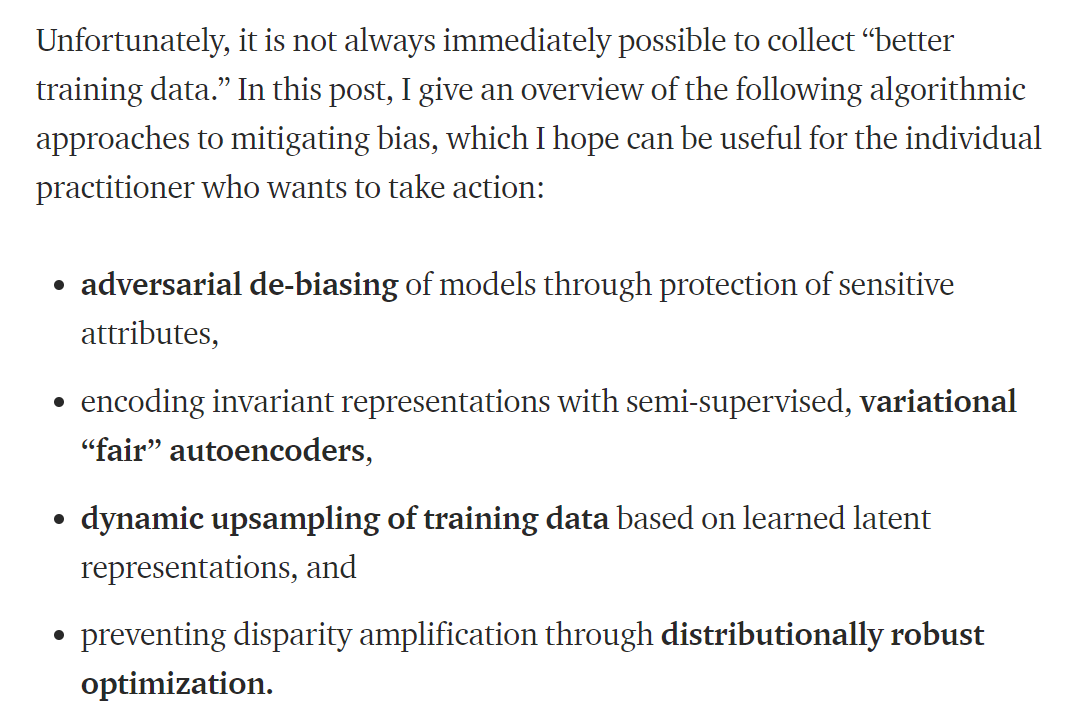
These are some of the many examples showing that the computer science community is now actively engaged with the issue of lacking objectivity in systems where it has been (and often still is) implicit. Interestingly, the approach tends to be one of searching for ways to regain a status of objectivity, or semblance thereof. The lack of objectivity is a fault in the training data, rather than in the algorithms.
Beyond the specific case of algorithms, objectivity associated with information/knowledge production/distribution is understood as a means of (and to) legitimacy and power. Foucault’s work on power and his own terms like governmentality have been influential for discussion on the discursive regimes that bring about legitimacy. This is the case in research on algorithms alike; Foucault is often cited in discussions that deal with the issue of algorithmic objectivity, governance and the associated processes of legitimation.
Building on Foucault then, scholars have explored the ways in which algorithms are implicated in mechanisms of power. One dimension of this concerns the use of knowledge and information as a means through which certain realities become habitual, unquestioned. Beer (2017) stresses the need to understand algorithms as constituent parts of a knowledge apparatus through which power is enacted; through both their form and applications, algorithms promote (the value of) their calculations and establish a need for ‘knowledge-based governance’. Kitchin and Dodge (2011) highlight algorithms’ role in discursive regimes, which sustain and reproduce themselves through a set of socio-spatial conditions. Constituting discursive regimes, they persuade people to their logic by making their message common sense. In the context of broader socio-technical systems, the semblance of algorithmic objectivity can also be interpreted as a means of certain actors in maintaining their invisibility; for instance Facebook’s work as a curating agent (Rader & Gray, 2015). In these cases their ‘power’ is achieved through a process of self-legitimation of structures bigger than algorithms themselves, in which they are nonetheless inextricably implicated.
What might be seen as a particular mechanism of this, is Gillespie’s (2014) elaboration on the algorithm as a “technology of the self” (Foucault 1988 in Gillespie, 2014). As such, algorithms work to ‘independently ratify one's public visibility’ (Gillespie, 2014: pp), with the effect of giving people a reassuring sense of confirmation – of themselves, and of what they are doing. Confirming self-image and self-understanding is a compelling way to promote certain discourses and their embedded logics. In this sense, algorithms become external tools that people use for introspection. Aids to anchor and position themselves in social space. This begins to chip in to my interest in subjectivity, as it is through such tools that individuals formulate and revise their own subjectivity. In Foucault’s terms, technologies of the self are as important to guide individuals’ acts as ‘techniques of domination’, although they are nonetheless often – or always to a certain extent – ‘integrated into structures of coercion and domination’ (1993: 203).
Following another line of argument, the objectivity and legitimacy of algorithms can be seen as a set of interdependent pragmatic beliefs. Morozov (2011) suggests that the guise of objectivity may be a way of dealing with the weight of the responsibility of gatekeeping information, especially in a context where the complexity of information systems is far beyond the comprehension of any single individual; the unit we know how to attribute accountability in. Drawing parallels with domains like journalism, the notion of objectivity can also be seen as a ritual in tasks that involve complex ethical considerations (Gillespie, 2014; Dorr & Hollnbuchner, 2017). Natale and Pasulka (2019) further elaborate that this can be viewed as a sort of pragmatic religious experience, where the assumption of objectivity – its myth – is an underlying element of the belief in algorithms’ legitimacy. Just like functions, code or labels on output data stated in natural language describe what a machine is supposed to be understood to be doing rather than naming their exact activity, a certain level of pragmatism is needed to avoid paralysis and go with it. If it works, it works – and the ‘it’ is easier left objective.
As the examples I’ve discussed here imply, algorithmic objectivity is contested and people have moved on past articulating this point, to thinking about the reasons why and to what ends the myth stands, how the issue could be ‘fixed’, or how people go about it in their daily interactions involving algorithmic agents.
If not objective, then what?
With the criticism of algorithmic objectivity stated, it becomes relevant to think about what these agents are instead. Positive definitions and conceptualisations are of course numerous and build on the criticism of algorithmic objectivity to different extents and in different ways. As I’ve elaborated on above, some replace algorithmic objectivity with the notion of objectivity as a public performance; a constituent part of contemporary power mechanisms and governance tools. Gillespie (2014) describes this as a process of logonomic control, in which users bestow tools with legitimacy, which carries over to the information provided, and – most importantly – to the provider by proxy too. Natale and Pasulka (2019) consider it a collectively held imagination of a desirable future. Others conceive of algorithms as sociotechnical assemblages or imaginaries (for example Jasanoff & Kim, 2015; Kitchin, 2017), or processes unfolding in time and space in our daily lives (Kitchin & Dodge, 2011). These works drift further away from positioning the myth of objectivity at their core. The open question this poses is suddenly as broad as: what are algorithms, given that they are not objective? So instead of addressing this broad speculation, I would like to develop a single alternative to objectivity here, namely subjectivity.
Criticised for not being objective, algorithms have come to be labelled as biased. Algorithmic objectivity becomes algorithmic bias – a term now widely used in both academia and practice. What makes bias the better choice of antonym to objectivity when dealing with algorithmic agents? My issue with the notion of bias is that it (1) safeguards the objectivity of the algorithm by relaying the ‘problem’ to its training data, its makers, society, or others around it except it-self and (2) greatly reduces the complexity of a heavy, nuanced concept like objectivity to something much narrower, with the connotations of a statistical term, or a euphemism for being unjust – a state that could be corrected with the right (counter-)weights. Instead, I would like to propose algorithmic subjectivity as a viable alternative to bias.
Knowledge (of the subject) in the context of digital media
Subjectivity is not a new concept to be relating to algorithms. Rather than being attributed to algorithmic agents themselves though, subjectivity in this domain has been explored as a human characteristic, embedded in discussions on the ways in which algorithms are transforming modes of knowledge. Before turning to subjectivity in specific, I begin by elaborating briefly on some of the epistemological implications of algorithms and digital media more generally; changes that have brought about a transformation in the role of the subject.
Intricately linked with and increasingly taking over responsibility for pretty much everything that has to do with information, digital media play an important role in today's knowledge and truths. The concept of truth, in this context, is being increasingly associated with actuality (Rouvroy & Stiegler, 2016). Speaking on the topic of algorithmic governmentality, Rouvroy (2016) argues that the ‘pure’ actuality that algorithms (seem to) give access to, figures as pure reality – where pure reality constitutes truth, or perhaps even superseeds it. Why stick to a concept like truth loaded with a certain level of absolutism, when we now have the tools to instantaneously access the here and now? What we understand as truth, in this sense, shifts from being grounded in things to being directly elicited by networks of data evolving in real-time.
As scholars like Kluitenberg (2008) have discussed in relation to the topic of temporality in the age of new media, the possibility of real-time undermines our as yet habitual understanding of the world in terms of the past-present-future; the historical progression of time through which knowledge is (or was once) made and consolidated. In real-time, things can be revisited at any instant when their consultation or assessment is needed. A priori assumptions can be dismissed as inaccurate, as in real-time a past state may no longer correspond to reality. The so-called virtual, then perhaps closer approaches the real through the merit of its actuality. In this sense, a real-time temporality admits the dynamism of things and events. With truth replaced by pure actuality, it begins to seem as though things are speaking for themselves (Rouvroy & Stiegler, 2016).
The absence of a mention of subjectivity stipulates its role in this reality; it is no longer needed to establish knowledge. Rouvroy (2016) argues that subjectivity, viewed as a potential source of uncertainty, is by-passed in a reality of algorithmic governmentality. Subjectivity here is primarily nested in evaluation. As human evaluation is replaced by automated processes, the evaluating subject becomes not only obsolete, but also most likely ‘incorrect’. By-passing the subject, I would add, is also a practical necessity in a regime where human reflexive subjects could simply not keep up with the real-time evaluative work that a regime of algorithmic governmentality demands. Rouvroy (2016) captures the core of this argument succinctly in her concluding remark, proposing that we have nothing to tell when fragments of data speak for themselves.
Taking Rouvroy’s position further, Fisher and Mehozay (2019) propose that digital media have brought about a new paradigm for seeing audiences of information. Digital media, compared to for example mass media, are not a new way of doing the same thing, but rather a completely different way and thing altogether. They term this paradigm the ‘algorithmic episteme’, which they primarily contrast to the ‘scientific episteme’. The scientific episteme, realised through for example mass media, is based on a regime of truth that is oriented backward in time and relies on subjective evaluation in its search for objectivity – very much in line with the temporality contrasted to real-time above. The algorithmic episteme is instead a future-oriented regime of anticipation in which objectivity can be found in behaviour itself. Although commenting specifically on human behaviour, the argument here is the same as Rouvrouy’s (2016), namely that this behaviour now speaks for itself.
Removing the human subject
Fisher and Mehozay’s (2019) algorithmic episteme then, is another way of arriving in a regime where subjectivity is by-passed. To me it is interesting that in focusing on audiences (who become individual ‘users’), Fisher and Mehozay step away from situating their arguments in abstract Foucauldian concepts like governmentality towards an analysis in which the epistemological implications to and for the subject are central. Their argument is grounded in three socio-technical features of digital media, that together have a transformative effect on our epistemology; user-generated data, inter-connected platforms and algorithms. In digital media, the primary source of knowledge about audiences comes from user-generated data, then processed by algorithms to anticipate future behaviour and trends. User-generated data, in this context, have been cleverly described by Lupton (2016) as ‘lively’, in that they are constantly flowing and founded on ‘life itself’. Data represent the most mundane and often trivial features of humanness; correspondingly seen as ‘lively’, this helps found the objectivity of behaviour, and behaviour, therefore, as a valid source of knowledge – one that can best be processed by algorithms.
Algorithms are not interested in the ‘essence’ of things, or of our behaviour for that matter; as Fisher and Mehozay state, ‘successfully seeing an audience is predictive, rather than explanatory’ (2019: 11). As we find computational methods that are virtually unlimited in the number of variables that they can process, the need for controlling variables and for supervised learning is reducing. Presumed variables are therefore gradually becoming redundant and essentialist judgements are no longer productive in knowing one’s or another’s self (Fisher & Mehozay, 2019). Broad discrete categories like ‘gender’, ‘sex’, or ‘race’ that were once at the core of our public-facing subjective identities, are no longer productive or informative, as an algorithm can process countless other measures of our behaviour that are more telling in predicting our future behaviour. Likewise, the popular notion of the ‘quantified self’ becomes a way of knowing one’s own subjectivity (Lupton 2016; Neff & Nafus, 2016 in Fisher & Mehozay, 2019). Beyond changing the public presentation of individuals’ subjectivity, the algorithmic episteme introduces a new way of understanding oneself by mobilising ways of knowing offered by digital media; it creates a new type of knowledge about the self for the self, based on looking at what the data say, rather than searching for explanatory depth.
In the context of the algorithmic episteme, knowing the self is achieved without subjectivity. When subjectivity is formulated as the alternative to the objectivity of algorithms, analysis of Foucault’s work is once again relevant to this discussion. Fisher (2020) argues that the tripartite link between the self, media and knowledge that Foucault theorised as the core of Western modernity no longer requires participation of the self – of the subject. Fisher (2020) elaborates on this from an interesting historical perspective that highlights the role of technologies like writing in creating subjectivity. Practices like diary writing or confession created spaces in which the self could be objectified in the world, and self-consciousness could grow. Viewed as technologies of the self, diaries were at first a means to establish subjectivity, and later to continuously improve the self through self-expression, self-reflection, self-improvement. Building on a number of more detailed examples, Fisher (2020) states that media facilitate(d) the knowledge necessary to attain an emancipated self; subjectivity itself is (/was) a bridge to emancipation through gaining the necessary knowledge. Until this point the argument is primarily an analysis of Foucault’s work, but rephrasing the statement in the past tense is what gives room to a new regime in which the quest for self-expression/ -reflection/ -improvement no longer yields emancipatory knowledge. In other words, coming to grips with your emotional state by carefully reconsidering your week in a diary entry gives you little insight into the reason why certain articles make it to your suggested reads.
Algorithmic subjectivity
The key idea that the works discussed in the previous section boil down to is that algorithms limit or, ultimately, erase the subject – in both, the subject’s self-awareness and in our understanding of what constitutes knowledge more broadly. But what if subjectivity can be had by a non-human agent? I would suggest instead then, that subjectivity is not erased from the equation, but simply displaced to the algorithm.
In his discussion of Google’s leaked video on its possible near-future aspirations entitled ‘The Selfish Ledger’ (Savov, 2018), Fisher (2020) comes perhaps closest to a case in which a subjectivity-imbued algorithm is not too far from an (imagined) reality. The video was put together by Nick Foster, head of design at Google’s X and a co-founder of their Near Future Laboratory, and David Murphy, a senior UX Engineer at Google. Put very succinctly, it imagines a future in which a ledger (used as a metaphor for a data-collecting technology) comes to represent each individual and begins to guide their user in ways that are in line with their personal best interests. The video draws on Lamarckian evolutionary theory, suggesting the possibility of a ledger outliving its user. It makes parallels between DNA as the code of organisms and behaviour as the code of behaviour – framed, as I have discussed above, as today’s real-time source of objectivity. Overall the video is perhaps best described as a thought experiment on the role big data could play in Google’s notion of a good life.
I would like to stress that Fisher’s (2020) analysis is situated in the framework of the algorithmic episteme (Fisher & Mehozay, 2019); algorithms here, do not simply bring a new way of doing old things, but rather necessitate, or bring with them, a new epistemology – a new definition of what it means to ‘know’. Google, in Fisher’s terms, is the ‘single most important in articulating, defining, advocating, and working towards realising’ this new epistemology (Fisher, 2020:18). This makes the analysis of materials that guide or showcase these ideas particularly valuable to making sense of concepts like today’s ‘algorithmic imaginary’ (Bucher, 2017); Fisher argues that ‘The Selfish Ledger’ pitches a vision of ‘knowledge without subjectivity’ for the near future.
Commenting on the concluding section of Google’s video Fisher writes:
“(6) Volition – the last and most far reaching tenet of the epistemology underlying the selfish ledger is the idea of knowledge without a human subjectivity. This is encapsulated in the metaphorical attribution of volition to the ledger, as it being selfish. The ledger has a will to know, it seeks always to get more data. And if relevant data is missing, the ledger can elicit an action with the user in order to obtain it.” (2020: 10)
The character of the ledger in the video, its intentional and ultimately self-interested behaviour as well as Fisher’s evaluation of it as manifesting ‘volition’, suggests to me that the ledger becomes a subjective agent in the course of the imaginary’s progression. Rather than simply making subjectivity redundant, the ledger assumes this formerly human role/quality itself. Implicit processes like self-reflection or self-improvement are now carried out computationally, or through what Gillepsie (2014) terms ‘calculations’.
Looking into algorithmic subjectivity further
Perhaps the primary issue with allowing algorithmic agents subjectivity, is dealing with the question of consciousness. Just as Fisher (2020) explains that the widespread availability of cheap paper in the 16th century led to a massive growth in self-consciousness as individuals could formulate and develop their subjectivity through writing, today’s increasing availability of computational resources could fulfill the same function for algorithmic agents. Indeed if processes like self-reflection can be carried out by the ledger computationally, they could simultaneously open up the room for (self-)consciousness – at least this is the way in which human subjectivity is formulated and further theorised (for example Habermas, 1991 in Fisher, 2020). I would like to avoid this question of consciousness here as it is one that has been dealt with repeatedly in considerable depth since thought experiments like the Turing test, and with a renewed vivacity since the 90s by figures like Dennett (1990), when AI slowly started taking off. Perhaps though, thinking subjectivity, without an explicit need to deal with consciousness – partly as it has and is being dealt with elsewhere – could be a relevant way to make sense of algorithms that admittedly lack objectivity.
Drawing parallels with other contested characteristics of machinic agents could be useful in further elaborating on this issue. The possibility of algorithmic subjectivity, for example, echoes the issue of computational creativity. Much like subjectivity, creativity is viewed as a feature of (human) intelligence. In Boden’s (2004) terms, it is implicated in the association of ideas, reminding, perception, analogical thinking, searching a structured problem-space, and reflective self-criticism. Although by now algorithms have been able to demonstrate creativity across the different categories of it that people like Boden (1998; 2004) have formulated, ascribing them the quality of creativity remains a grey area. A reason for this may be the argument that creativity implies a positive evaluation; faced with negative evaluation based on prejudice, or simply disinterest, the quality of creativity cannot be granted. I would suggest that subjectivity likewise requires a positive evaluation from the perspective of the one granting it.
Conclusion
Attributing subjectivity to certain algorithms makes the intelligence in AI more challenging to our own. Endowed with its own volition, Google’s metaphorical ledger becomes its own thinking subject. This may suggest that the epistemologically transformative nature of digital media is overstated by scholars like Rouvroy, Fisher and Mehozay; perhaps subjectivity is not by-passed, or made obsolete in our knowledge systems, but rather outsourced to non-human agents. The intellectual credit that this would endow algorithms with by extension, makes such arguments difficult, and potentially problematic to formulate (particularly in light of the decades of discussion on issues like machinic consciousness, which remain unresolved). The capacity of self-reflection and access to the tools that actuate it, could be seen as extending a ‘technology of the self’ to an algorithmic agent – rather than the algorithm being simply a technology of the self to its user. This of course poses questions that are equally challenging to come to terms with as the myth of objectivity itself. How can subjectivity be contained and taken responsibility for, if it is held by a socio-technical actor – one that outdoes any single individual in its complexity? In this light, I view the narrow, technical notion of ‘algorithmic bias’ as equally pragmatic to simply assuming ‘algorithmic objectivity’ for the sake of its utility. A further inquiry into the possibility of algorithmic subjectivity could be one way to understand knowledge with and through algorithms in more depth.
References
- Beer, D. (2017). ‘The social power of algorithms’, Information, Communication & Society, 20(1): 1-13, DOI: 10.1080/1369118X.2016.1216147
- Bucher, T. (2017). ‘The algorithmic imaginary: exploring the ordinary affects of Facebook algorithms’, Information, Communication & Society, 20(1), 30-44, DOI: 10.1080/1369118X.2016.1154086
- Dennett, D. C. (1990), ‘Can Machines Think?’, In: Kurzweil, R., The Age of Intelligent Machines, MIT Press.
- Dörr, K. N., & Hollnbuchner, K. (2017). ‘Ethical challenges of algorithmic journalism’. Digital journalism, 5(4): 404-419.
- Fisher, E., Mehozay, Y. (2019). ‘How Algorithms See Their Audience: Media Epistems and the Changing Conception of the Individual’, Media, Culture, and Society, 41(8): 1176–1191.
- Fisher, E. (2020). ‘The ledger and the diary: algorithmic knowledge and subjectivity’. Continuum, 1-18.
- Foucault, M. (1993). ‘About the Beginning of the Hermeneutics of the Self: Two Lectures at Dartmouth.’ Political Theory, 21(2): 200–227.
- Gillespie, T. (2014). ‘The relevance of algorithms’. In: T. Gillespie, P. Boczkowski, & K. Foot (Eds.), Media technologies: Essays on communication, materiality, and society (pp. 167–194). Cambridge, MA: MIT Press.
- Kitchin, R., & Dodge, M. (2011). Code/space: Software and everyday life. Cambridge, MA: MIT Press.
- Kluitenberg, E. (2008). Delusive Spaces. Essays on Culture, Media and Technology, Rotterdam: NAi Publishers & Amsterdam: Institute of Network Cultures.
- Lupton, D. (2016). The Quantified Self. Malden, MA: Polity Press.
- Mackenzie, A. (2015). ‘The production of prediction: what does machine learning want?’, European Journal of Cultural Studies, 18(4–5): 429–445.
- Morozov, E. (2011). "Don't be evil." The New Republic, July 13. Retrieved 15 March 2020 from http://www.tnr.com/article/books/magazine/91916/google-schmidt-obama-gates-technocrats.
- Natale, S., & Pasulka, D. (Eds.). (2019). Believing in bits: Digital media and the supernatural. Oxford University Press.
- Rader, E., & Gray, R. (2015). ‘Understanding user beliefs about algorithmic curation in the Facebook news feed’. In: CHI’15 proceedings of the 33rd annual ACM conference on human factors in computing systems (pp. 173–182). New York: ACM. Retrieved from http://dl.acm.org/citation.cfm?id=2702174
- Rouvroy, A., & B. Stiegler. (2016). ‘The Digital Regime of Truth: From the Algorithmic Governmentality to a New Rule of Law’, Online Journal of Philosophy, 3: 6–29.
- Savov, V. (2018). “Google’s Selfish Ledger Is an Unsettling Vision of Silicon Valley Social Engineering.” The Verge, May 17, Retrieved 15 March 2020 from https://www.theverge.com/2018/5/17/17344250/google-x-selfishledger-video-data-privacy
- Xu, Joyce. (2019). “Algorithmic Solutions to Algorithmic Bias: A Technical Guide”, towards data science, June 18. Retrieved 15 March 2020 from https://towardsdatascience.com/algorithmic-solutions-to-algorithmic-bias-aef59eaf6565.