MYTH/ Between Myth, Rumours and Expert Algorithmic Knowledge
“What I didn't realize at the time was that I was developing a formula to beat the algorithm.” (Rachel Paul, teacher of the ‘Beat the Algorithm’ course)

With this text I explore some of the ways in which scholars make sense of non-scholarly and non-technical conceptions of algorithms. First, I review some of the approaches that researchers have taken in gaining insight into how users understand and relate to their respective platforms of choice. This imaginary-driven end of algorithmic research often focuses on users’ lack of technical or expert knowledge of the algorithms in question. Drawing on Bishop’s (2019) research, I find it relevant to acknowledge that algorithmic knowledge of some working in professions that rely on algorithms may be expert level within their domain. In this context, I discuss my own attempt to make sense of the Instagram algorithm through a number of articles sharing strategies for visibility management on the platform.
Making sense of an invisible other
Folk theories, folk science, beliefs, dreams, gossip, myth; these are some of the ways in which predominantly non-technical, non-expert ways of understanding are labelled and theorised. As research under the broad umbrella of algorithmic knowledge production states, understanding algorithms is a nuanced task that involves users and spaces far outside the domain of computer science. Algorithms are sociotechnical assemblages according to many (for example: Kitchin & Dodge, 2011; Gillespie, 2014; Kitchin, 2017), which in part implies that they are enmeshed in a co-production and the blurring of lines between real and imagined realities (Jasanoff, 2015). Indeed Bucher’s (2017) often cited concept of the ‘algorithmic imaginary’ stresses just how important ordinary affects and cultural imaginaries are in shaping how algorithms are understood and used.
The focus is on daily life, everyday interactions. How do users make sense of an invisible and intangible other, that figures so prominently in their daily lives? Encounters with algorithms become tangible in the spaces, where they can be met (Bucher, 2017; Kitchin & Dodge, 2011). Qualitative methods like interviews, surveys or content analysis have been used by researchers to gain access to these meeting points and the imaginaries that they shape. Bucher (2017) for example, studied peoples’ personal stories about Facebook, Eslami et al. (2015) researched users’ perceptions of the Facebook News Feed curation algorithm, DeVito, Gergle and Birnholtz (2017) analysed folk theories surrounding Twitter’s algorithmic curation.
Concepts like ‘folk theory’ (DeVito, Gergle and Birnholtz, 2017), ‘intuitive theory’ (Rader & Gray, 2015), ‘belief’ (Natale & Pasulka, 2019) or ‘myth’ (Mosco, 2015) are used to qualify and unpack these sources of algorithmic knowledge production. Drawing on Legare and Gelman (2011) for example, DeVito, Gergle and Birnholtz understand folk theories as ‘contextual frames through which users interact with a system’ (2017: 3165); this grounds algorithmic understanding in causal models of how algorithms might work, as well as in opinions and attitudes about how they might operate, and ‘abstract theories’ more generally. Similarly, Eslami et al. 's (2016) list of tools used to reason about the invisible consists of sketching, analogy and comparing assumptions to outcomes.
Beliefs and myths are, in this sense, integral to managing everyday interactions with algorithms. Natale and Pasulka (2019) point out that although computational technologies may have been intended to be perceived as rational, they are often readily associated with things like mind reading or miracles instead, which echo the supernatural. Especially as today’s digital technologies open new ways to rethink ancient mythical dreams like that of immortality, or make use of impossible metaphors like ‘cloud computing’ promising an unattainable networked interconnectivity, many assumptions about algorithms are shaped by the desires their makers and users have of them. I would like to put some extra emphasis on including the makers here, as thinking past folk theories along the lines of myths and beliefs opens up this ‘non-technical’ approach to those who do have technical expertise in the domain of algorithms.
As shared assumptions become public truths, the performance, in turn, goes both ways. As though not interesting enough to study without qualification, researchers tend to stress that user beliefs shape the system. Rader and Gray (2015) position user beliefs about the Facebook curation algorithm as an important component in the feedback loop that shapes it. Likewise Bucher (2017) notes that the algorithmic imaginary is ‘not just productive of different moods and sensations, but play[s] a generative role in moulding the Facebook algorithm itself’ (2017: 12). The learning and evolving nature of the machine is underlined.
The 2020 Instagram Algorithm
To think about the ways in which people make sense of algorithms I thought my time would be best spent looking outside of academic accounts. I read 20 articles from 2019 and 2020 detailing tips on how to gain and manage visibility on Instagram, including all associated comments and number of external links. These articles were the top 20 I found searching Google for the query ‘beat the algorithm’ that focused on Instagram. I skipped a few of the results which were about different platforms (there were one or two about LinkedIn and one about Twitter; the rest were all about Instagram, even though I did not include it explicitly in my search). The texts were predominantly written by professional content creators/ influencers, artists or small business owners using Instagram for promotion, agencies or individuals selling Instagram related products, bloggers and social media experts.
I was interested in the popular notion of ‘beating the algorithm’ as in the context of a particular platform, it narrows the writers and readers down to people who want to use it for (self-set and -evaluated) success. The articles were all aimed at content creators, most leaning towards the professional end of the spectrum; users who use the platform seriously and invest their time, often with the (prospective) aim of monetising the work they put into it. Information about platform visibility is often unavailable to content creators – primarily for reasons of inaccessibility and corporate secrecy. To manage the visibility that their professions rely on, what Bishop (2019) terms ‘algorithmic gossip’ becomes an important method and resource for gaining knowledge.
Bishop (2019) also points to the binary nature of the way in which algorithmic knowledge production tends to be studied. One end of the research examines how algorithms are constructed in the sites that hold sanctioned expertise and technical knowledge – tech companies, for example. The other, looks at daily-life interactions with algorithms, highlighting how algorithms can be made sense of despite lacking technical knowledge or expertise (like the studies of Rader & Gray, 2015, Eslami et al., 2015., Bucher, 2017, DeVito, Gergle & Birnholtz, 2017) This leaves little room for those, whose work is dependent on algorithmic knowledge, even though their occupations do not qualify them as technical or expert on the subject matter. Bishop’s own study focuses on the example of beauty vloggers ‘whose feminised output positions them outside of the technical, yet whose work is contingent on algorithmic visibility’ (2019: 2950). Instagram’s content creators fit a similar category; although reliant on algorithms by the nature of their profession, their domain knowledge excludes them from expert status when it comes to algorithms.
Algorithmic gossip circulated by those who meet and rely on an algorithm in their work on a daily basis is therefore a relevant (and sometimes only publicly available) source for their understanding. Bishop (2019) compares algorithmic gossip to a form of reverse engineering; one of the methods that Kitchin (2017) lists, as a way to study algorithms, similar to Seaver’s (2013) notion of ‘revealing’ algorithms. Meticulously described in flashy click-bait articles and reiterated in their comment threads, discussions on the Instagram algorithm cycle through the themes of business models, monetisation, metrics, bot-like behaviour, consumption of time, scheduling, commitment, enthusiasm, encouragement, struggle, outsmarting machines, subjectivity, care, community, nostalgia, victimisation or frustration.
My research is presented in the form of a fictional text that deals with some of these issues.
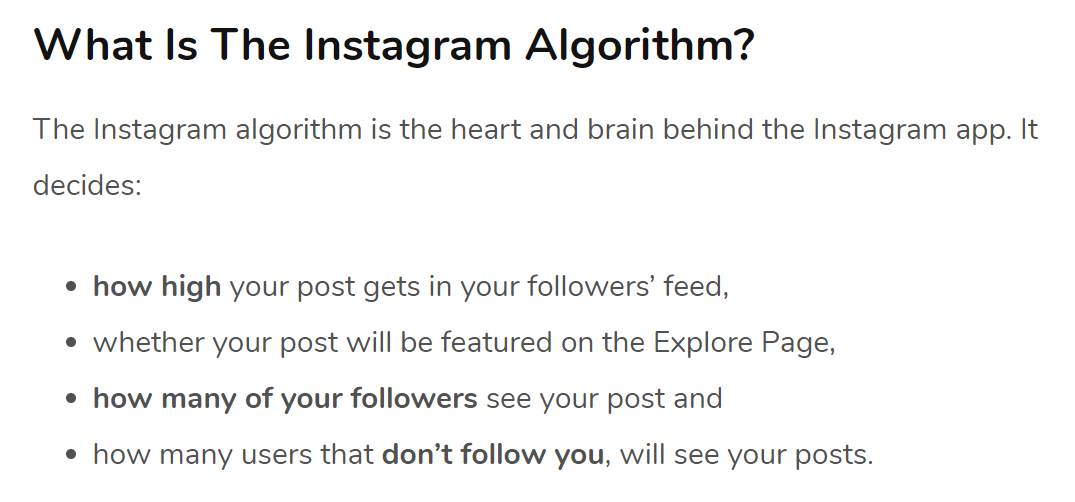


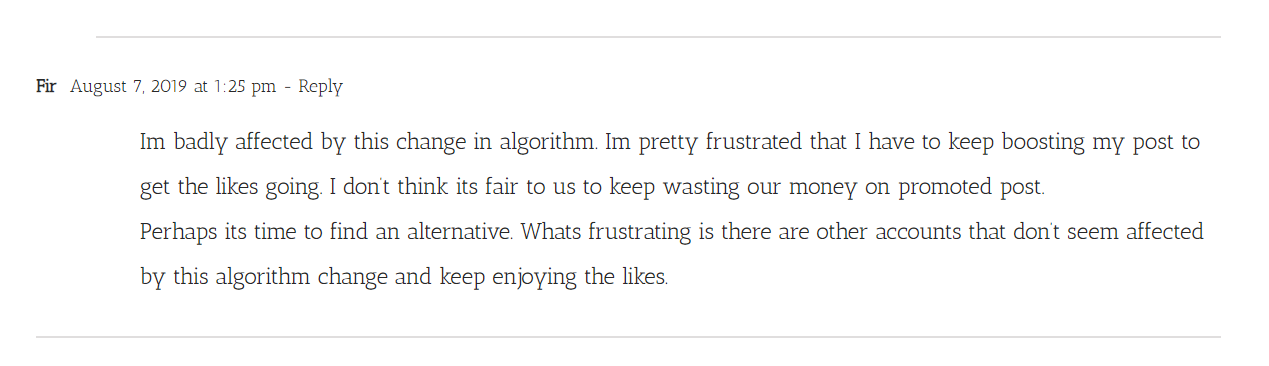
Content, the King
Let me share some of my secrets with you. I know, I know, they don’t come in as easy as they used to. The good old days. But we’re here and now and let’s make the most of it. We can still make it work. Really it’s all about engaging. Being authentic, and real. Today content is king. Authentic, yes, that’s the right word. But don’t say it too often. Never reuse the same hash in too many posts – you wouldn’t want to be mistaken for a bot. Organic reach is what we're after here. 7’s the right number. Some say 5, but I think those are just lies. Just not enough, or like you put too much thought into it. Like you spent hours studying the size chart. S, M, L, XL – who cares? You might want to hit some of those trending ones from time to time. Get on the wave. 30’s clear spam though. Steer clear. No one’s out there arguing that one. Repeat and reuse – yes – to keep them engaged – but keep the rhythm real. Be an active user. How else do you think you can be taken seriously? It’s a sin to buy followers, but no sin to steal a meme. That’s what they’re for. Use them, people really love to engage with them. They feel connected. They show that you’re down-to-Earth. It’s a chip in your part in the two-fold process. You’re you after all, and sometimes that’s just the way you feel. People want to see, hear, but especially see you. Share community. Not everything’s perfect, neither does it have to be. People want to be reminded they’re not alone. So respond, like, follow, DM – show them you’re interested, that you care. Others will follow. Care back. But wait a minute. Within 60 mins is usually about right. Don’t like it straight away. Easy. And follow back after 24 hrs, or – if you can wait – 48 hrs. Hate those follow unfollow types. They really messed things up here. A part of the problem. Like this you can filter them out sometimes. Keep engaging with only those who are really interested. And only reciprocate when it’s good stuff. It’s all about the content after all. What if I just don’t find it relevant? Sometimes the colour grading is just off. Doesn’t sit with me, doesn’t draw me in. I don’t want to be seeing more of that. Content is king. Would hate for my followers to see that. My interactive friends. I love speaking to them. I carousel my content for them – they love it. Save it for later even, and you should remember to do the same. We always use more than three words in comments – icons alone might not count. And you should show you’re interested after all. Long captions, lifelong. They have so many insights. Only they can tell you what they really want to see. More stuff, new stuff, authentic stuff. That’s what I’m talking about. Know your audience. Give them a little treat. Be regular, reliable. Scheduling is key. Punctuality helps too (but remember, stay relaxed, keep it playful). If you usually post Monday, Tuesday and Saturday, then stick to it. That’s when people will learn to check for new stuff. But it’s not enough, let’s not kid ourselves. At least once a day is the minimum. Reaching only 10% of your followers since the update last summer, two posts gives you access to at least 20%. That’s still not a lot I know, but hey – see it as an opportunity. You can get more intimate. The people who see your stuff are more likely to care. It’s not just chronological. A way to manage the boom. So many. Too many? It keeps the scrolling endless – but first things first. There’s no getting around it. And relevance makes it first place. Use the features. They're there for a reason. Record videos, yes – not just images! Let your camera stitch those frames together. Make you move and really bring you to life. Put up stories. Not sure it makes a difference, but whatever – just do it. That’s what they say. That’s what I think anyway. Take up time and keep them looking. Eyeballs glued to their screens. Thumbs keep scrolling. Geotag popular destinations. Only if you’re really there though. Make it interesting. Selfie shots are so personal. They let you keep it raw and tell a story. You. Your brand. Go live. Who said you need a professional? It takes time, yes, but you’ll get the hang of it. Everyone can beat the algorithm. We can do it. Consider a scheduling tool and some solid analytics. Some are even free, and decent too. Whatever they say, there’s really no difference between business and private. The exposure’s the same. It’s just an account. Those are just rumours. People circulate lots of rumours. Read the facts, trust the data. Keep on top of it. Study, learn and experiment. It really is a science. I’m just sharing some friendly advice. Spend hours on rumours. I’m repeating myself, but this one’s really important. I hope this meets others out there who have suffered from the latest version. Just like me. I completely relate. You really need to understand it. A tough transition. I know it’s always changing, so we can’t really put a finger on it. Outsmart full-circle, get ahead of the curve. Some tricks do work though and I can tell you from first hand experience that it takes serious commitment. It’s not like in the old days. We’ve got to work hard. Really stand out, by keeping it real. Great content. It can be so much fun once you get the hang of it. Get over the long-gone vanity metrics. Can’t even see them anymore, but likes still filter. That was a big blow. They matter. Keep liking. At first it’s so frustrating. So many sleepless nights. Tonight I hope you’ll sleep well though, with these new insights. I’m finally starting to get the algorithm.
Subscribe for weekly tips and updates.
References
- Bishop, S. (2019). Managing visibility on YouTube through algorithmic gossip. new media & society, 21(11-12), 2589-2606.
- Bucher, T. (2017). ‘The algorithmic imaginary: exploring the ordinary affects of Facebook algorithms’, Information, Communication & Society, 20(1), 30-44, DOI: 10.1080/1369118X.2016.1154086
- DeVito, M. A., Gergle, D., & Birnholtz, J. (2017). Algorithms ruin everything:# RIPTwitter, folk theories, and resistance to algorithmic change in social media. In Proceedings of the 2017 CHI Conference on Human Factors in Computing Systems (pp. 3163-3174). ACM.
- Eslami, M., Karahalios, K., Sandvig, C., Vaccaro, K., Rickman, A., Hamilton, K., & Kirlik, A. (2016). First i like it, then i hide it: Folk theories of social feeds. In Proceedings of the 2016 cHI conference on human factors in computing systems (pp. 2371-2382). ACM.
- Gillespie, T. (2014). ‘The relevance of algorithms’. In: T. Gillespie, P. Boczkowski, & K. Foot (Eds.), Media technologies: Essays on communication, materiality, and society (pp. 167–194). Cambridge, MA: MIT Press.
- Jasanoff, S., & Kim, S. H. (Eds.). (2015). Dreamscapes of modernity: Sociotechnical imaginaries and the fabrication of power. University of Chicago Press.
- Kitchin, R., & Dodge, M. (2011). Code/space: Software and everyday life. Cambridge, MA: MIT Press.
- Kitchin, R. (2017). Thinking critically about and researching algorithms. Information, Communication & Society, 20(1), 14-29.
- Mosco, V. (2005). The digital sublime: Myth, power, and cyberspace. Mit Press.
- Natale, S., & Pasulka, D. (Eds.). (2019). Believing in bits: Digital media and the supernatural. Oxford University Press.
- Rader, E., & Gray, R. (2015). ‘Understanding user beliefs about algorithmic curation in the Facebook news feed’. In: CHI’15 proceedings of the 33rd annual ACM conference on human factors in computing systems (pp. 173–182). New York: ACM. Retrieved from http://dl.acm.org/citation.cfm?id=2702174
- Seaver, N. (2013). Knowing Algorithms. Media in Transition 8, Cambridge, MA. Retrieved February 10, 2020, from http://nickseaver.net/papers/seaverMiT8.pdf